Addressing Data Center Capacity Challenges Amidst AI Expansion
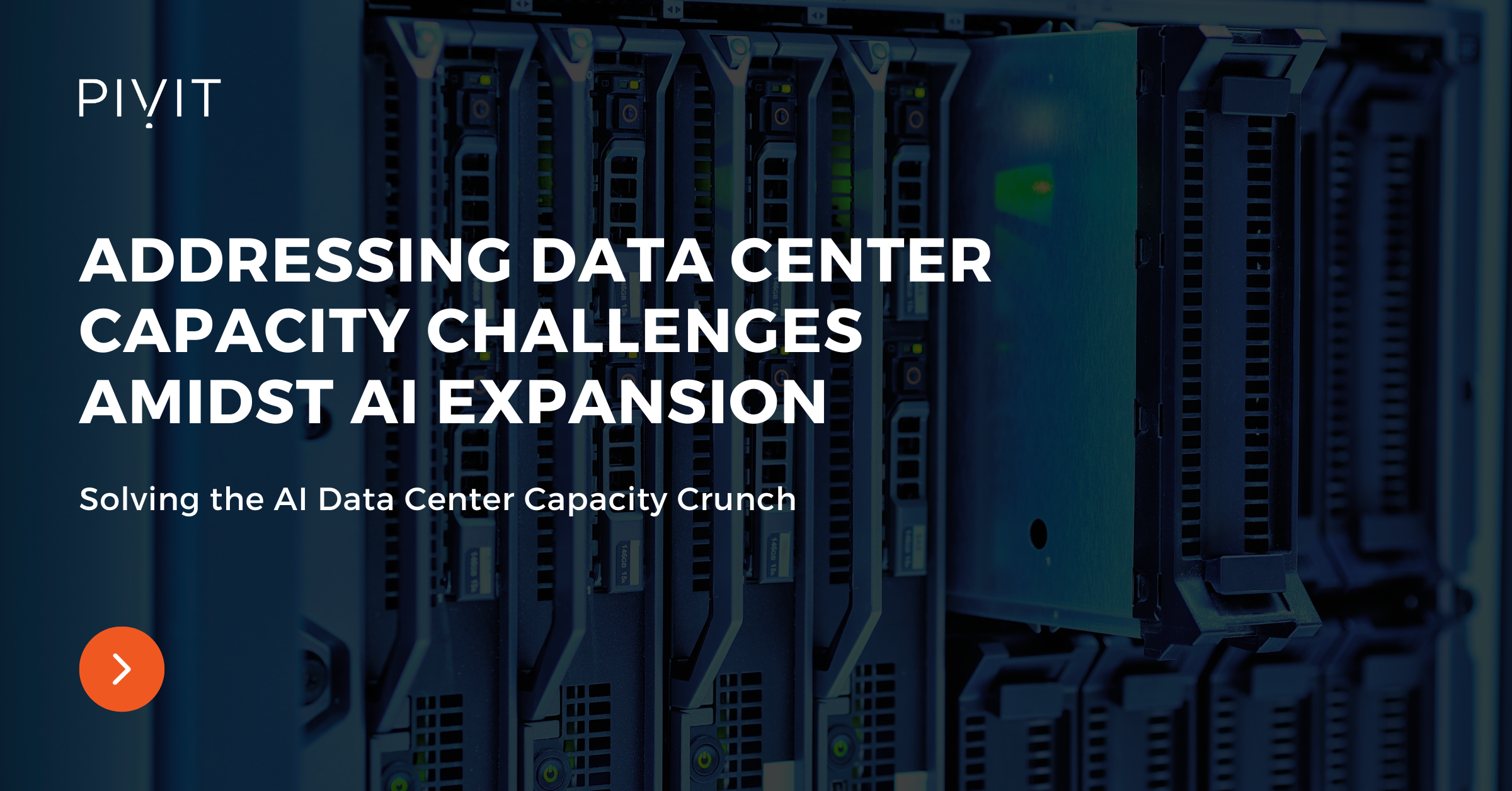
Data center space and power consumption doubled between 2010 and 2020. Despite the enormous growth, mainly on the back of cloud computing, there’s a growing capacity shortage. Artificial Intelligence (AI), the most sought-after technology at the moment, is increasing demand for data center space—capacity that simply isn’t there.
While AI isn’t necessarily the new kid on the block, recent developments have kickstarted the race for adoption. That means more enterprises directly working with AI. And that’s creating a strong demand for data centers to power those, often computing and energy-intensive, AI workloads.
All players, at every level, are vying for data center space. The lack could create hurdles to these innovative technologies' rapid expansion and adoption. Then, there’s the concern about the impact on the grid that new data centers and AI workloads will have and the associated carbon footprint.
Soaring Demand for AI Data Centers
There are challenges and opportunities stemming from the increased capacity demands, but one thing is clear—the data center industry is on the cusp of exponential growth. Take, for example, the estimates on the growth of modular data centers. It’s estimated to grow at a CAGR of 19% to a whopping $65 billion by 2027. On the other hand, hyperscalers have even higher estimated growth with a CAGR of 27.9% (poised to reach $1,529 billion).
While these estimates are somewhat general, the most relevant numbers on data center demand due to AI come from McKinsey. According to the consulting firm, the demand for data center capacity may increase by 19-22% from 2023 to 2030. In terms of energy demand, those numbers translate to 171 to 219 gigawatts (GW). It also says that demand could rise by 27% or 298 GW in the rather more ambitious scenario. The baseline is just 60 GW.
The estimates on power demand from data centers put into perspective just how big the AI revolution is going to be. However, the current situation within the data center spaces raises concerns about whether the supply will even meet this increasing demand. That, essentially, puts the whole AI dream, so to speak, in the balance.
The estimates from McKinsey, although helpful, may not be entirely accurate. The firm itself talks about the difficulty of predicting capacity demands, “Estimating the precise size of that deficit is hard because of uncertainties surrounding the pace of rising demand, the extent to which innovations might improve power efficiency, and limited knowledge concerning the longer-term expansion plans of data center owners and operators.”
Where Is Data Center Capacity Shortage Acute?
The deficit between rising demand and the current supply of data center capacity isn’t uniform across different regions. Some regions have more severe shortages than others.
Europe, in particular, is seeing shortages in data center capacity for AI workloads despite having an overall increase of 22% in the last year. The region risks getting behind in the AI revolution because of this lack of data center capacity. Shortage of space and increasing pressure on the grid are the likely reasons behind the slow pace of data center growth relative to other regions.
The traditional data center hubs like Frankfurt, London, and Dublin are struggling the most in terms of expanding capacity. As a result, growth is shifting to other cities like Berlin and Warsaw.
North America, in comparison, has been quick to address the growing demand. Data center construction in 2024 increased by 69%, particularly in primary markets. However, long lead times for equipment and power constraints are delaying construction.
In the Asia-Pacific market, big players in the data center industry are increasing capacity with new constructions. STACK, a data center developer, is working on projects in markets like Japan, Malaysia, and Australia, indicating that some progress is being made to cater to the growing demand in the most populous region in the world.
Who Is Leading the AI Data Center Capacity Demand?
Unsurprisingly, hyperscalers like Amazon Web Services (AWS), Microsoft Azure, Google Cloud, and Baidu are leading the AI-centric data center capacity demand. That’s primarily because all these big tech companies are working on their AI projects and host other leading AI companies. For instance, OpenAI uses Google Cloud, which is backed by Microsoft.
Other companies that are reusing and modifying the models from these tech giants are also relying on the private cloud for resources.
McKinsey predicts that by 2030, most of the AI workloads will be hosted by hyperscalers and cloud service providers. It estimates that 60-65% of the workload will be cloud-hosted, while the remaining will be hosted privately.
The Main Challenges with Data Center Expansions
So, what exactly is creating hurdles in meeting the growing data center capacity? First, expanding existing data centers and building new ones takes time. These often multi-billion dollar projects can take years, from planning to design to approval to construction. Although many are already under construction, the demand is increasing faster than new data centers are (and can be) built.
Grid Limitations
A more significant challenge in the growth of data centers is the availability of grid power, particularly in primary markets that are already supersaturated. The addition of huge facilities to already densely populated mega centers like London or San Francisco can potentially put a toll on the local grid.
According to BCG, the power demand from data centers will grow to 130 GW by 2028, increasing every year by 16%. But can the grid accommodate the massive power surge? That’s a different story.
AI is infamously power-hungry, which has led to experts predicting exponentially high energy usage. However, recent developments in China, particularly the development of the DeepSeek model, are now raising questions about energy efficiency. DeepSeek’s open-source generative AI model is supposedly cost-effective and energy-efficient. There’s some indication that AI workloads may eventually be made less power-hungry and costly. Still, with the growing adoption of AI overall, even energy-efficient models will require more data center space.
Increasing Regulations for Data Centers/Environmental Impact
Another challenge for companies investing in data centers and those building them is the regulation regarding their performance. With such a huge power draw, data centers are a key component of the carbon footprint in many regions. In places like Europe and Singapore, data centers may need to go through the sustainability hoop.
Quick expansions while also offsetting emissions can be tricky.
What’s the Solution?
The data center capacity issue is very real, one that’s slowly unraveling as more companies take on missions to make the next big thing in AI. It’s a solvable problem, though.
1. New Locations
Traditional data center hubs are becoming increasingly saturated. To alleviate this pressure, organizations must explore new, geographically diverse locations. This includes canvassing for regions rich in renewable energy sources (hydro, wind, solar), even if they are more remote.
Consider areas with cooler climates to reduce cooling costs. Prioritize locations with robust fiber optic connectivity or plans for its expansion.
This strategic diversification can unlock new capacity and reduce reliance on congested hubs, ensuring long-term scalability.
2. Design Improvements
Modern data center design must prioritize efficiency and environmental sustainability. Implement advanced cooling technologies like liquid immersion and direct-to-chip cooling to manage the high heat densities generated by AI workloads. Optimize airflow and utilize free cooling when possible.
Embrace modular designs for rapid deployment and scalability. Integrate renewable energy sources and implement smart energy management systems to reduce carbon footprint.
3. Better Infrastructure
AI workloads demand high-performance computing (HPC) infrastructure. This requires significant upgrades to existing infrastructure. Invest in high-bandwidth interconnects and low-latency networks to facilitate rapid data transfer between servers. Deploy specialized hardware such as GPUs, TPUs, and FPGAs to accelerate AI processing.
The solution lies in software-defined infrastructure, which enables dynamic resource allocation and orchestration. Strengthen power distribution and backup systems to ensure uninterrupted operation. Integrate advanced monitoring and management tools to identify and address potential bottlenecks proactively.
4. Collaboration
A driver behind the very AI revolution that’s causing capacity shortages is collaboration. And that can also be the same solution.
No single organization can shoulder the entire burden of AI expansion. Strategic collaboration is essential. Partner with colocation providers to access additional capacity and expertise. Establish partnerships with smaller regional data centers to distribute workloads and reduce reliance on hyperscale facilities. Explore edge computing solutions to process data closer to the source, reducing latency and bandwidth requirements. Develop data-sharing agreements with other organizations to pool resources and expertise.
The Time Is Now!
The big tech players, non-profits, and governments will need to come together to make data center expansion fast and sustainable to meet the growing demand from AI developments and, at the same time, keep the environmental impact in check.
Even AI itself can be used to find remarkable solutions. It won’t be easy, but it’s not impossible either.