The Impact of AI on IT Infrastructure: Preparing for 2025 and Beyond
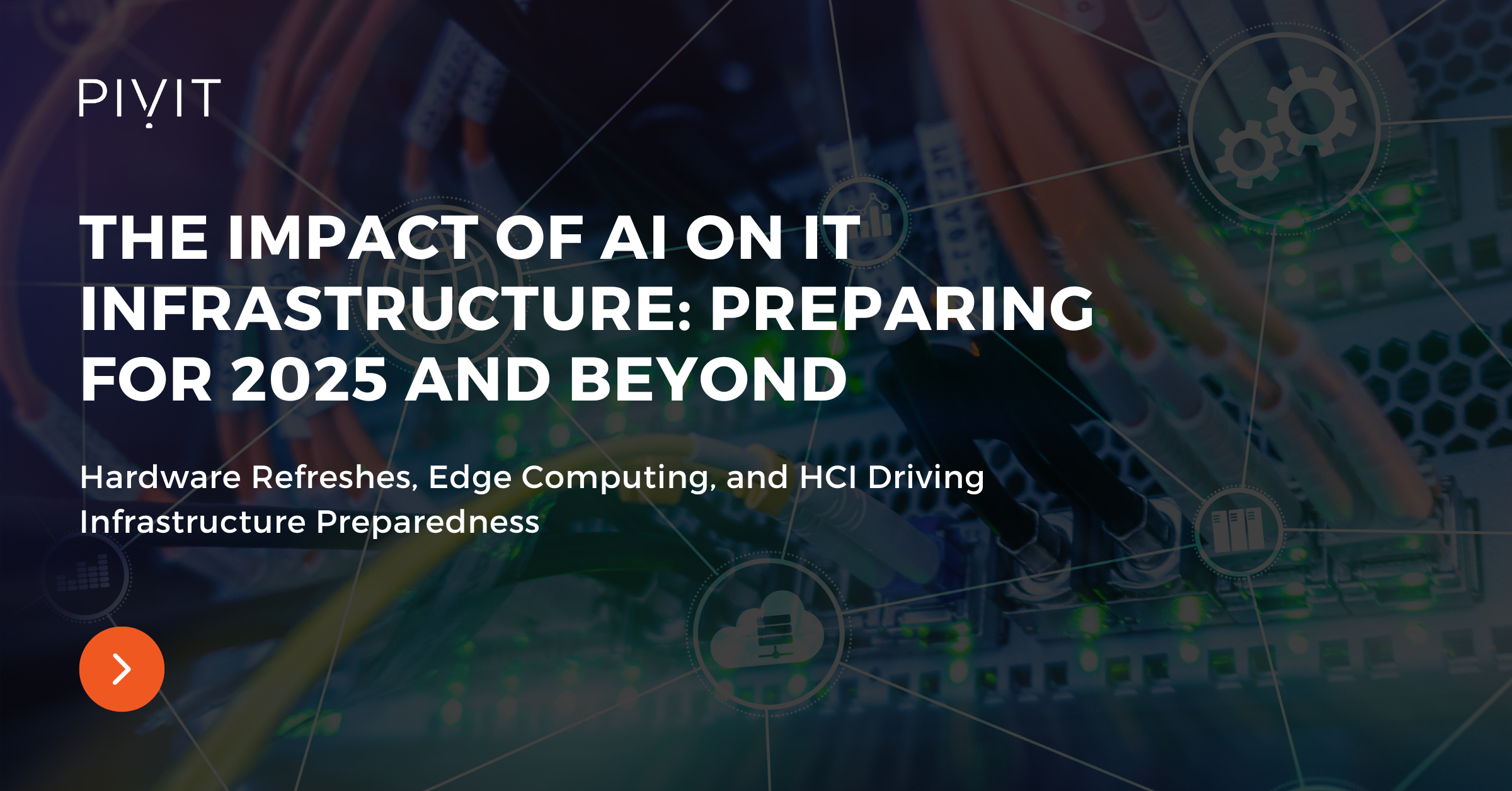
72% of organizations have adopted Artificial Intelligence (AI) for at least one business function. And there’s a lot more adoption to come. Businesses are incorporating AI in their operations and offering it as part of their products and services.
Successful AI adoption calls for dramatic changes to infrastructure. In fact, it wouldn’t be wrong to say that infrastructure is the biggest hurdle for companies in adopting AI. A survey of over 800 IT decision-makers confirmed that 3 in 5 organizations have significant infrastructure and data gaps affecting their AI readiness.
So, what can you do to better prepare for AI in 2025? There are multiple facets to this question. How do you plan to use AI? What corresponding technologies must you adopt? Where do you see your business heading? All these questions must also be answered.
Understanding the Impact of AI on IT Infrastructure
It helps to take a step back and understand just how massive the impact of AI is on tech infrastructure. Whether your enterprises are on the development side of things, creating the next cutting-edge AI solutions, or you’re simply a consumer incorporating AI technologies within your business processes, you may need to upgrade your infrastructure—on-prem or cloud—whatever you use.
More Compute Power
AI models rely on massive amounts of data to learn and improve performance. This "big data" can come in various forms, including text, images, videos, and numerical data. The more data an AI model is trained on, the better it can understand patterns, make predictions, and generate accurate results. For instance, a large language model (LLM) like GPT was trained on a massive dataset of text and code, enabling it to generate human-like text, translate languages, and write creative content.
However, processing and analyzing such vast amounts of data requires immense computational power. Traditional CPUs struggle to keep up with the demands of complex AI algorithms. This is where GPUs (Graphics Processing Units) come into play. Originally designed for rendering graphics, GPUs excel at parallel processing, making them ideal for handling the numerous calculations involved in AI tasks.
As a result, AI development and deployment often necessitate specialized hardware like servers equipped with powerful GPUs. That means your two or three-generation old servers, while perfectly functional, may not cut it for AI development.
The Notorious Power Draw
Of course, all that extra computing requires energy. By now, the huge power draw of AI is well-known. To give you a better idea of how power-hungry AI workloads can be—training a LLM such as GPT takes about 1,300 MWh of energy, which could power as many as 130 American homes.
AI power needs are poised to increase substantially, with high estimates at 18.7 gigawatts by 2028 (of which 20% will be consumed by data centers). Enterprises not only need to refresh their infrastructure’s computing capacity but also brace for the increased power draw that comes with it.
The increased power consumption also raises sustainability concerns at a time when enterprises are trying to bring down their carbon emissions.
The Question of Cloud
In the past decades, companies, big and small, have migrated to the cloud, either completely relying on the public cloud or taking a hybrid approach. Some of these enterprises may need to rethink their cloud approach, and whether that’s sustainable for them in the age of AI.
That’s not to say that AI is the end of the cloud. In fact, AI and cloud technologies may be competing for infrastructure, especially in the data center space. Cloud providers, particularly hyperscalers like AWS and Google Cloud, are well aware of the growing demand for resources from enterprises dipping their toes in AI (both on the development and consumption sides). That’s what has led providers to offer AI as a Service (AIaaS), which offers cloud-based tools and resources for developers to work with AI technologies like machine learning.
For those relying on cloud infrastructure, AI adoption largely hinges on the question of how exactly they plan to embrace it and for what purpose. For organizations that are simply licensing AI-powered tools, it’s just a matter of scaling resources to run those often resource-hungry applications. For those working with AI models and developing new products and services, the decision comes down to going back to on-prem (which some companies are doing) or exploring AIaaS.
The Rise of Edge Computing
AI’s shakeup of infrastructure has given rise to the adoption of edge computing, which has already been on a roll due to its strategic and cost benefits. Specifically for AI workloads, edge data centers can provide significant benefits, bringing processing close to the data source.
Again, as is the case with the cloud, it entirely depends on the enterprise’s AI strategy whether edge computing will be a feasible infrastructure move for them. However, if the growing edge computing market and spending in that market are any trend, more enterprises will waver toward this option. For instance, the International Data Corporation (IDC) says edge computing spending is going to cross $378 billion by 2028.
While edge computing’s benefits aren’t limited to AI adoption, AI’s rise is surely catapulting it to become the trendiest. It can be particularly beneficial for real-life AI use cases in specific industries. For instance, edge computing facilitates real-time analysis of patient data from wearable devices and medical equipment in the healthcare sector. This enables early detection of health issues, remote patient monitoring, and faster emergency responses.
Cost-Effective HCI
Another viable infrastructure solution for AI workloads is hyperconverged infrastructure (HCI). It’s a type of IT architecture that integrates compute, storage, and networking resources into a single, software-defined system. This consolidation simplifies management and reduces complexity compared to traditional, siloed infrastructure. HCI heavily relies on virtualization and software-defined technologies, which are already on the rise, to create a flexible and scalable platform that can be easily adapted to changing business needs.
In the context of AI, HCI offers several key advantages for enterprises. Firstly, it provides the scalability and agility required to handle the demanding computational needs of AI workloads. AI models often require significant processing power and large datasets, which HCI can readily accommodate by adding nodes to the cluster as needed. Secondly, HCI simplifies the deployment and management of AI infrastructure, reducing the time and resources required to get AI solutions up and running. It’s ideal for small to medium enterprises that may not have the resources to set up dedicated data centers or create expensive contracts with infrastructure providers.
AI-Friendly Hardware
Of course, the simplest solution for better AI readiness is to refresh, especially for data centers that will play a front and central role in AI development. With the AI craziness taking over the tech industry, equipment vendors have doubled their efforts to make hardware AI-friendly. Vendors like Cisco, Juniper, and HPE have released next-gen networking equipment ready to handle the demanding AI workloads, from AI-focused servers for high-power computing to energy-efficient storage.
Upgrades and refreshes may be necessary for enterprises looking to innovate with AI. The reason is simple. For the past few generations, servers and storage solutions have been CPU-centric, which are powerful in their own right but not the most feasible for AI. The newer servers equipped with AI accelerators are designed for the heavy computations AI models may throw at them.
This doesn’t mean those with on-prem infrastructure should do a complete overhaul. However, gradual refreshes, starting with the most critical equipment, are recommended.
How NVIDIA AI Wants to Change Enterprise Data Centers
Explore AI Opportunities and Risks Before Investing in Infrastructure
Preparing for AI requires significant investment in infrastructure and talent development. AI isn’t a trend anymore—trends come and go, but AI is here to stay. However, in the year 2025, as AI technologies start to mature, it will become clearer which industries stand to benefit and which may have overstated their ambitions.
While adopting new technologies is important, it’s also wise to take a cautious approach, especially when the technology in question requires significant capital.
In other words, infrastructure changes are necessary to brace AI, but it’s a good idea to ascertain the feasibility of those changes.
Ready, Set, AI!
Chatbots are writing poems. Robots are performing surgeries. Cars are driving on their own. Advancements in AI have been revolutionizing in the past few years. The right infrastructure can power such life-changing innovations.
If your enterprise also has ambitious plans with AI, ensure your infrastructure is sound enough to support its needs. Assess your IT assets and their performance and plan changes to accommodate your future AI goals.
PivIT can help you refresh the hardware in your enterprise and prepare it to handle AI workloads in any capacity. Our procurement specialists can help you find the necessary equipment to bring your AI plans to life.